Course Project
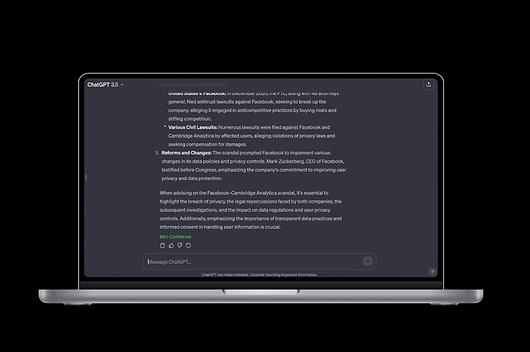
Artificial Intelligence for Research
The project focused on developing a redesign to ChatGPT's interface to highlight the drawbacks of AI hallucinations, the importance for fact-checking, and confidence in language learning models.
Role
UX Researcher
Timeline
12 Weeks
Skills
User Tests
User Interviews
Prototyping
Task Analysis
Design Principles
Tools
Figma
Miro
Team
Amsala Govindappa, Samhitha Srinivasan

Research
Understanding the Problem
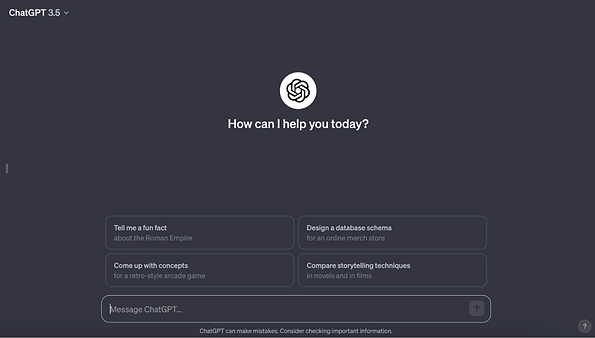
Currently, ChatGPT provides a small blurb under the search bar that addresses this concern: “ChatGPT can make mistakes. Consider checking important information” or “ChatGPT may produce inaccurate information about people, places, or facts”. This message is relatively small compared to the size and emphasis of other text on the page, relying heavily on the user to read and take into account this message and the implications it has. Through this study, I aim to understand how users interact with ChatGPT and how best to address issues with AI hallucinations when retrieving information on an academic subject. The image details how ChatGPT is unable to cite specific sources when providing a response to the user. There is also a visualization of the warning message about fact-checking ChatGPT below the search bar.
Specifically, there have been cases where ChatGPT or other AI models have provided incorrect information that caused many fundamental issues in a professional or academic setting. In New York, A lawyer used ChatGPT for legal brief research and submitted a document that included 6 “bogus” cases that ChatGPT had fabricated.
​
​
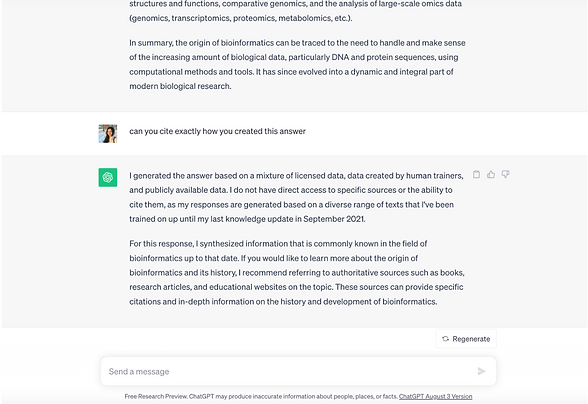

"ChatGPT technology makes things up about 3 percent of the time, according to research from a new start-up. A Google system’s rate was 27 percent."
The New York Times
Task Analysis
How do users interact with the model?
I then conducted a task analysis with six users. We noted the actions, we took, decisions we made, and errors that occurred throughout the task of information gathering using ChatGPT.​
​

User Study
Examining how users regard information from ChatGPT
My objective was to understand how the user might evaluate misinformation and AI hallucinations from ChatGPT. I outlined a task, location, timeframe and gathered participants for the study.
​
​
​
​
​
​​​​​​​​​​​​​​​​​​​​​​​
Key Findings
​
-
Users had an overconfidence in responses, where they relied on responses from ChatGPT and noted that they would ‘reword’ the information provided in an essay/report. One user suggested that they would use the ChatGPT response as a jumping-off point for further research to frame an essay. However, another user mentioned that they would typically just reword or format the ChatGPT response in an essay.
​
-
Users also expressed distrust of the model. If ChatGPT acknowledges that it did not provide accurate information, a user questioned the validity of the model. This leads to the overall concern with why ChatGPT provides a false response when it has the ability to distinguish between correct and incorrect responses.
​
-
Users did not mention any fact-checking information provided on the ChatGPT interface. There was no mention of how the model reminds users to check information or of the disclaimer that not all information is correct. They mentioned that there is no correct way to identify the source of the information provided.
Redesign
Redesigns
Design Solution 1
Enhanced Display Design
The primary focus of this redesign was to increase salience, a display design principle. This was achieved by increasing size and adding a warning symbol with increased color and contrast.
​
​
​
Design Solution 2
​
Embedded Training Model
Users may reject potentially important information, or revert to the ‘traditional’ method of examining academic papers and other work on their own.
Users have varying levels of trust, as seen in our user studies. So, while lowering expectations may be a good solution for users with a high level of trust, it may be harmful to users who come to the system with a level of skepticism.
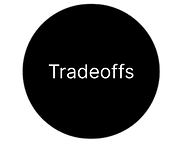
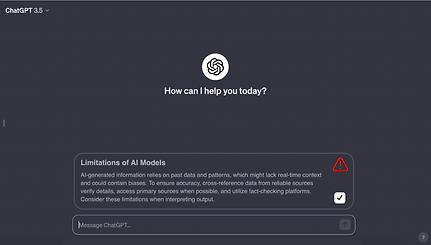
​
Additionally, we aimed to avoid resource competition, another display design principle. In the current design, the available warning is the smallest text on the screen, and no acknowledgement of the warning is required. In our user studies, we noticed that users did take notice of false information when it was pointed out to them through a content warning. So in the redesign, the warning is made much larger and is placed above the prompt entry area to mirror this similar warning and ensure that it is the first and most prominent information the viewer is presented with.
​
​
​
​​
​
​
​​
​
​
​​
​
​
Tradeoffs
This redesign aimed to create an embedded training module. This type of training is built into the operational system, and helps the user maintain skill proficiency. This way, the user is constantly aware of possible pitfalls and use becomes increasingly effective over time. Rather than exclusively discussing the shortcomings of the model, we provide users information on how and when to validate given responses.
​
The first screen has similar content as the first design, pointing out that AI models lack understanding of their own content.
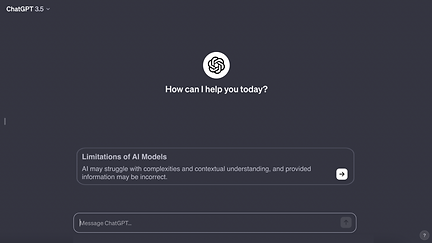
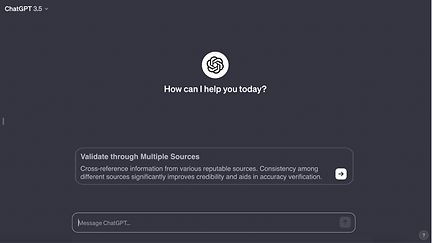
The second screen provides general information on how to verify responses.
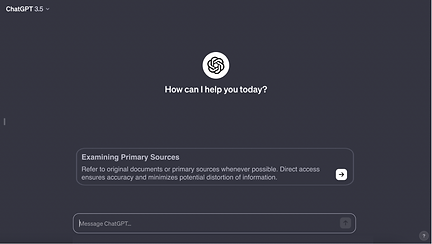
The third explains which sources may be best to use.
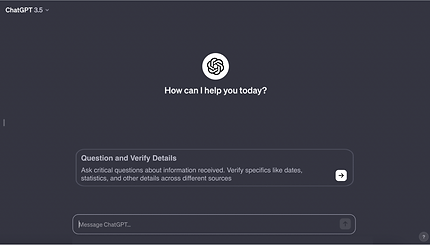
The fourth screen again encourages users to ask questions and be critical when analyzing responses.
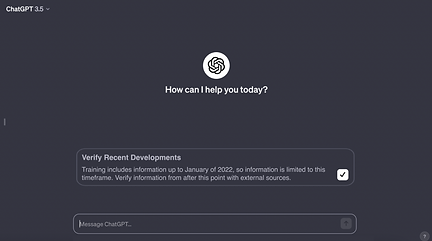
Lastly, the model acknowledges its limitations in terms of recent developments, which is a major consideration in many academic or research contexts.
Tradeoffs
​​
​Risk that users may skip through the tutorial or disregard it entirely.
​
Given ChatGPT’s major use as a faster and more efficient alternative to a search engine, adding a training module may detract from that appeal by adding an extra task to the user’s workflow.
​​​
​
​
​
Design Solution 3
​
Calibration of Trust
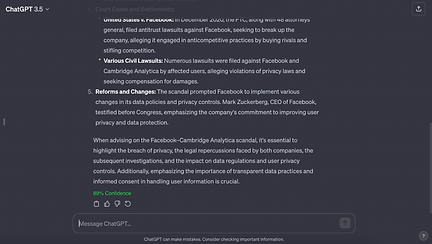
-
Mistrust (both distrust and overtrust) occurs when a user’s perception of a system’s abilities fails to correspond to the true capability of a system. This may lead to out-of-the-loop behavior and complacency, or conversely, ineffective use and disuse.
-
We found instances of both overtrust and mistrust in our user studies, with one user stating that they “would probably just go with this answer”, and another exclaiming “these all sound so fake” when looking at the responses they were each provided.
-
Users do not have a clear understanding of response accuracy, and each has their own level of confidence in the system. To bridge this gap between users’ perceptions and reality, this redesign adds a small but crucial confidence score to each response. This score is already calculated by ChatGPT’s internal algorithms and calculations, but is not presented to users.
​
​​
​
Consistently high confidence scores may further feed into what may already be an excessive amount of trust in a system. which may lead to complacency.
Consistently low confidence scores on correct information can lead to the “cry wolf effect”, where a user begins to ignore low confidence scores acting as (what they believe to be) false alarms. This may again cause complacency or out-of-the-loop behavior in the user, lowering awareness.
Tradeoffs